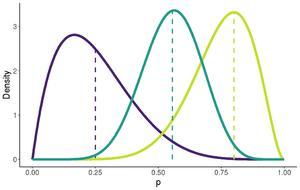
Bayesian Analysis of Binary Data
(0)Learn more about Bayesian inference, including prior specification and posterior analysis, for binary data using an interactive online resource.
Prerequisite Knowledge
- Be familiar with the probability concepts of a random variable and conditional probability.
- Know that the Bernoulli distribution (which corresponds to a Binomial distribution with n = 1) is used to model data that can only take two values, also known as binary data.
- Know that, when making inference for binary data, a random sample is used to estimate the value of the probability of success, p.
- Be able to calculate and interpret approximate confidence intervals for the probability of success of binary data.
- In the context of Bayesian statistics: 1) be familiar with the concepts of prior distribution, hyperparameter, likelihood, and posterior distribution; 2) know that the prior distribution should reflect your initial belief about the true value of the parameter; 3) be able to describe the roles of the hyperparameters in shaping the prior distribution
Learning Objectives
- In the context of binary data with success probability of p:
- Given data and a Beta prior distribution for p, calculate by hand the parameters of the posterior distribution of p.
- Given data and a Beta prior distribution for p, order, from largest to smallest, the prior mean of p, the sample mean, and the posterior mean of p.
- Given data and a Beta prior, obtain the posterior mean and variance of p using an online interactive resource.
- Compare and contrast the interpretation of a frequentist (1−α)×100% confidence interval for p with a Bayesian (1 − α) × 100% credible interval for p.
- Explain the impact that different prior distributions have on the posterior distribution.
Description
This resource is based on an interactive online web app (https://shiny-apps.stat.ubc.ca/FlexibleLearning/FirstBayes/Beta-Binomial/) developed at the University of British Columbia. The goal of the activity is for students to develop an intuition about Bayesian inference in the context of binary data, i.e., data that only takes two values, usually encoded as 0 (failure) or 1 (success). In the context of estimating the launch success rate of a space rocket, students first choose the conjugate Beta prior that best reflects their prior knowledge and uncertainty. Students then obtain and visualize (with the help of the web app) the posterior distribution, use it to compare frequentist confidence intervals with Bayesian credible intervals, and study posterior descriptive statistics. Finally, students apply their knowledge in an applied exercise.
Suggested Uses, Tips and Discoveries
This resource can be used to solidify students’ knowledge of Bayesian inference by doing an in-depth analysis of a specific example. Note that this resource should not be used as a first introduction to Bayesian inference since familiarity with the Bayesian parameter update process via Bayes’ theorem is a necessary prerequisite.
The activity sheet is appropriate for all class sizes and formats. It can be implemented in multiple ways—as an in-class activity, in a lab, as a homework assignment, amongst others. The activity takes around an hour to complete, but it can easily be split into parts if necessary. For example, Section 4 contains an applied exercise that can be left as a homework assignment to enhance students’ knowledge further. Section 3c contains optional theory-heavy exercises that can be omitted or left as a homework assignment as well.
Related Resources
Bayesian Analysis of Normal Data
Learn more about Bayesian inference, including prior specification and posterior analysis, for Normal data using an interactive online resource.
Creator
- Diluvi, Gian Carlo
- Lourenzutti, Rodolfo
Subject
Resource Type
Date Created
2021
Date Approved
Access
Everyone
https://statspace.elearning.ubc.ca/handle/123456789/403
Comments
Ratings in detail